Disruption Research at the 66th APS Division of Plasma Physics meeting
Great invited talks and SPARC contributions by the Disruptions team
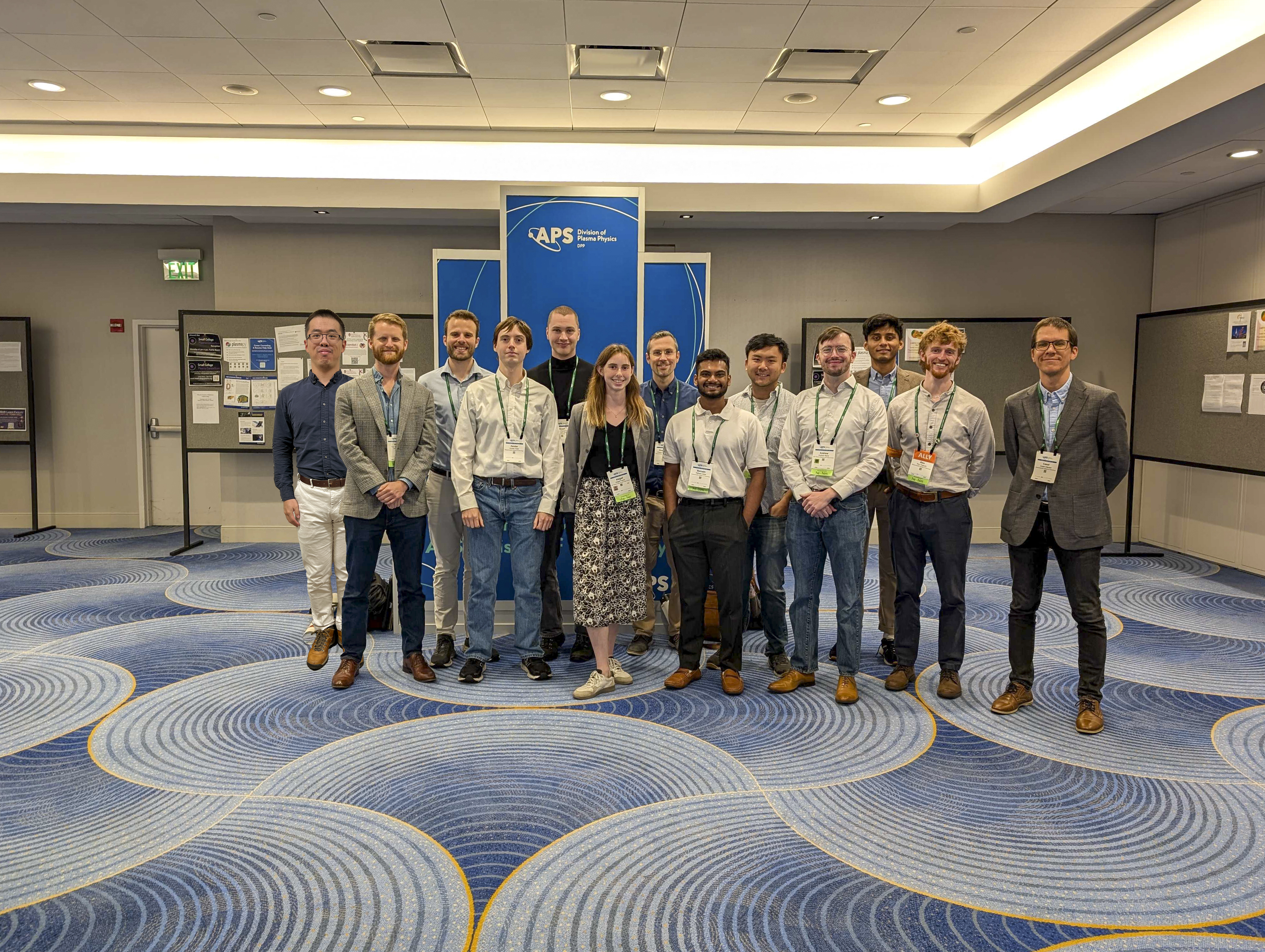
The 66th Annual Meeting of the APS Division of Plasma Physics took place in Atlanta, Georgia, from October 7th to 11th, 2024.
A significant contingent from the MIT PSFC Disruption Group actively participated in the conference, making valuable contributions through a range of oral and poster presentations, as well as 3 invited talks; 2 of which were given by senior graduate students.
In the MFE: Disruptions and MHD invited session, graduate students Andrew Maris and Ben Stein-Lubrano, as well as scientist Valerie Izzo, gave talks on multi-machine studies characterizing density limits, investigations of 3D affects on disruption mitigation efficiency on JET, and predictive modeling for mitigation on SPARC.
Throughout the MFE: High Field Tokamaks and MFE: Computational Science poster sessions, the disruptions group presented 10 posters, covering a wide range of topics from disruption prediction and consequences, to MHD stability, to disruption analysis software development. Many of these were targeted towards preparations for SPARC, focusing on fast-ion transport, runaway-electron/VDE consequences, and tearing mode detection, while others were more machine-agnostic, focusing on passive tearing stability, the disruptivity of UFOs, ramp-down optimization, and progress reports about the development of an Open and FAIR framework for disruption studies and Machine Learning applications. Of notable interest, the Disruptions Team has recently published open source DisruptionPy, a physics-based scientific framework for disruption analysis of fusion plasmas for AI/ML applications.
SPARC relevant talks were also presented during the MFE: High Field Tokamaks and the MFE: Machine Learning contributed oral sessions, reporting on the development of a Machine Learning-friendly simulation framework called POPSIM, an off-normal warning system for SPARC, and a surrogate model for calculating vertical stability.
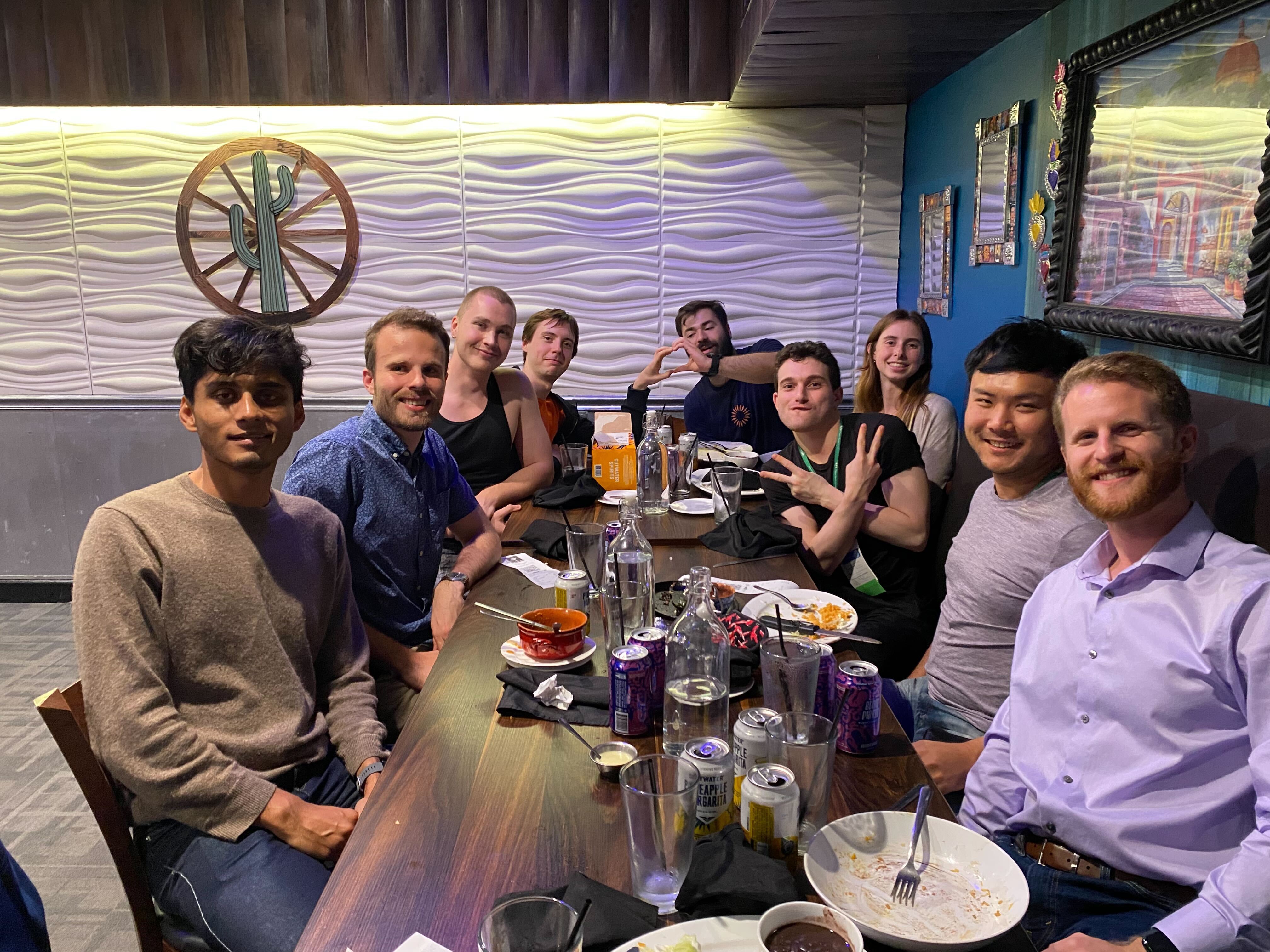
For more details on the various contributions, refer to the table below. The complete scientific program is available on the APS-DPP website.
Presenter | Type | Title | Session |
---|---|---|---|
A Maris | Invited | Correlation of the tokamak density limit with edge collisionality | BI02.2 |
B Stein-Lubrano | ” | Thermal energy mitigation and toroidal peaking effects in JET disruptions | BI02.3 |
V Izzo | ” | Predictive modeling for successful disruption mitigation on SPARC | BI02.6 |
A Wang | Oral | Plasma Operational Simulation (POPSIM): A Control-Oriented Simulation Toolbox for Parallel Simulation, System Identification, and Optimization | TO06.8 |
AR Saperstein | ” | Development and preliminary calibration of an off-normal warning system for SPARC | TO06.9 |
A Kumar | ” | Machine Learning model for real-time SPARC vertical stability observers | TO07.3 |
RA Tinguely | Poster | Fast ion driven instabilities, transport, and diagnostic opportunities in SPARC | NP12.101 |
JC Boguski | ” | High voltage insulation and operation of a runaway electron mitigation coil in SPARC | NP12.104 |
ZN Keith | ” | Development of an Observer for Neoclassical Tearing Modes in SPARC | NP12.107 |
SR Benjamin | ” | Simultaneous optimisation of tokamaks for passive tearing stability and fusion | NP12.108 |
C Clauser | ” | Modeling of SPARC Cold and Hot Vertical Displacement Events with M3D-C1 | NP12.109 |
H Wietfeldt | ” | Discerning Why Some High-Z UFOs in C-Mod Caused Immediate Disruptions while Others Did Not | NP12.111 |
A Feyrer | ” | Implementation of a runaway electron module in HEAT | NP12.112 |
A Wang | ” | Ramp-down Trajectory Optimization with Robustness to Physics Uncertainty with Reinforcement Learning Enabled by Scientific Machine Learning | NP12.126 |
GL Trevisan | ” | Functional Improvements and Technical Developments of a Community-driven and Physics-informed Numerical Library for Disruption Studies | PP12.9 |
Y Wei | ” | Physics validation of parameter methods in DisruptionPy | PP12.10 |
C Rea | ” | Open and FAIR Fusion for Machine Learning Applications | PP12.27 |
Enjoy Reading This Article?
Here are some more articles you might like to read next: