IAEA Workshop on AI for Accelerating Fusion and Plasma Science
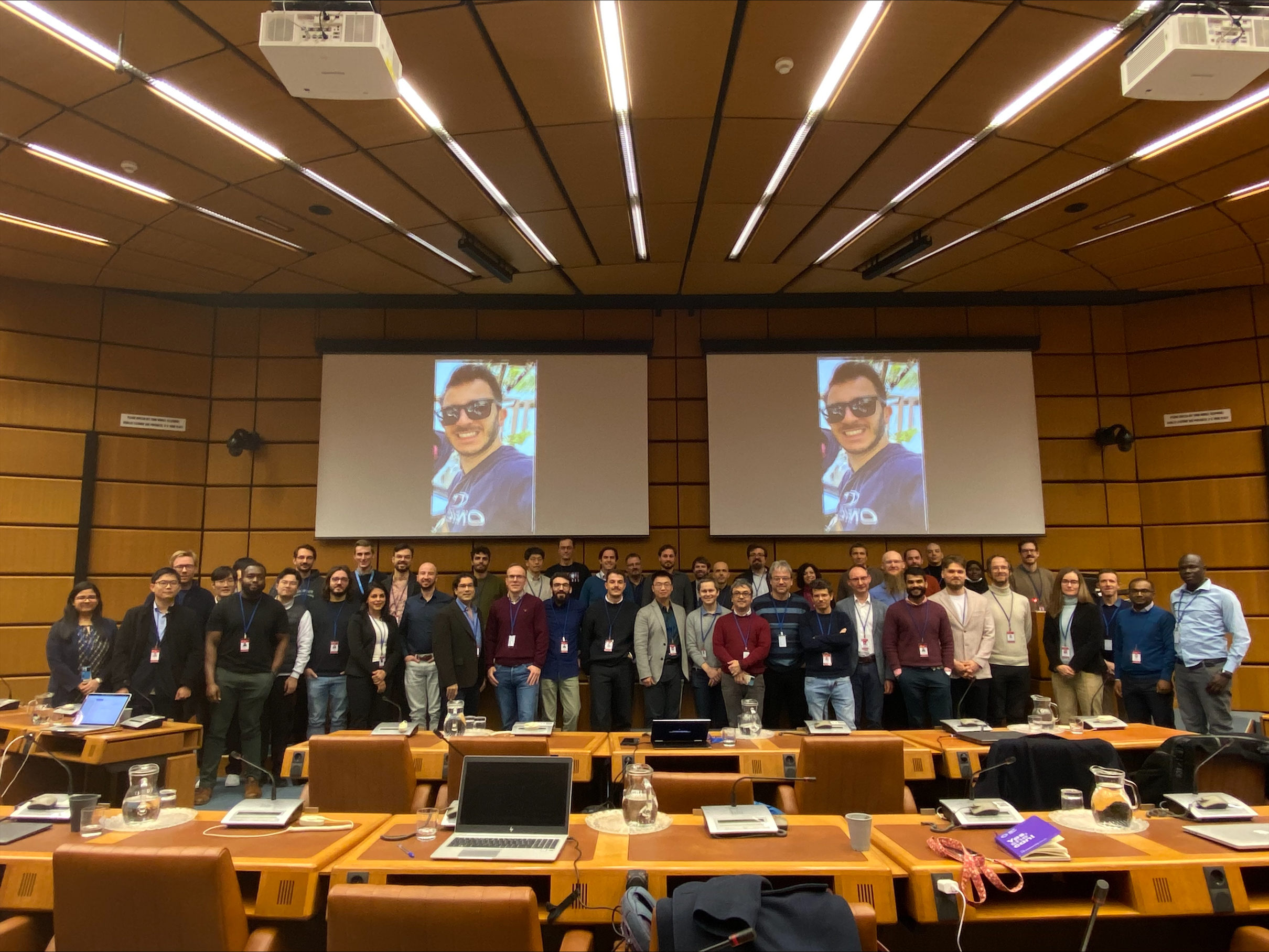
The first Workshop on AI for Accelerating Fusion and Plasma Science was held at IAEA Headquarters in Vienna, Austria, from November 28 to December 1, 2023.
The purpose of the event was to provide a platform for researchers, developers, practitioners, entrepreneurs and policymakers to discuss artificial intelligence applications to accelerate fusion and plasma science. An additional goal was to identify representative examples and related data to be shared through international collaboration, ideally leading to coordination or joint work within the IAEA.
The IAEA is currently sponsoring a Coordinated Research Project (CRP) on AI for Fusion with the goal of accelerating fusion R&D with AI, through the creation of a platform and cross-community network for innovation and partnership. Dr. Rea is leading the technical development of one of the CRP’s Work Packages, focused on increased data sharing and accessibility between international experimental facilities, under the guiding principles of open science and FAIR data.
With the help of a team of international scientists across magnetic and inertial confinement energy, Dr. Rea acted as Chair of the Programme Committee for the workshop, which spun off as a CRP activity.
The topics included dedicated sessions to current applications of Artificial Intelligence to Physics and Engineering fusion challenges, including advanced AI techniques for time-series prediction, self-supervised/unsupervised training on large datasets, and reinforcement learning for control and scenario design. One of the session centered around the topic of Enabling Infrastructure, where experts from the broader community were invited to discuss discuss how to accelerate fusion and plasma science leveraging High Performance Computing and AI resources.
Graduate student Allen Wang (MIT AeroAstro) presented a poster titled “Reinforcement Learning for Rampdown Scenario Design and Active Disruption Avoidance” and was furthermore invited to talk about “Large Language Models as Tools for Searching and Explaining Tokamak Shot Logs”, a collaborative project between Princeton University, Carnegie Mellon University and MIT students to leverage extensive textual data from experimental logbook entries by employing Retrieval-Augmented Generation (RAG) with state-of-the-art large language models (LLMs) to create chatbot instances that can answer questions using knowledge recorded in these historical text logs.
Enjoy Reading This Article?
Here are some more articles you might like to read next: